Google’s AI-First Strategy Brings Vector Support To Cloud Databases
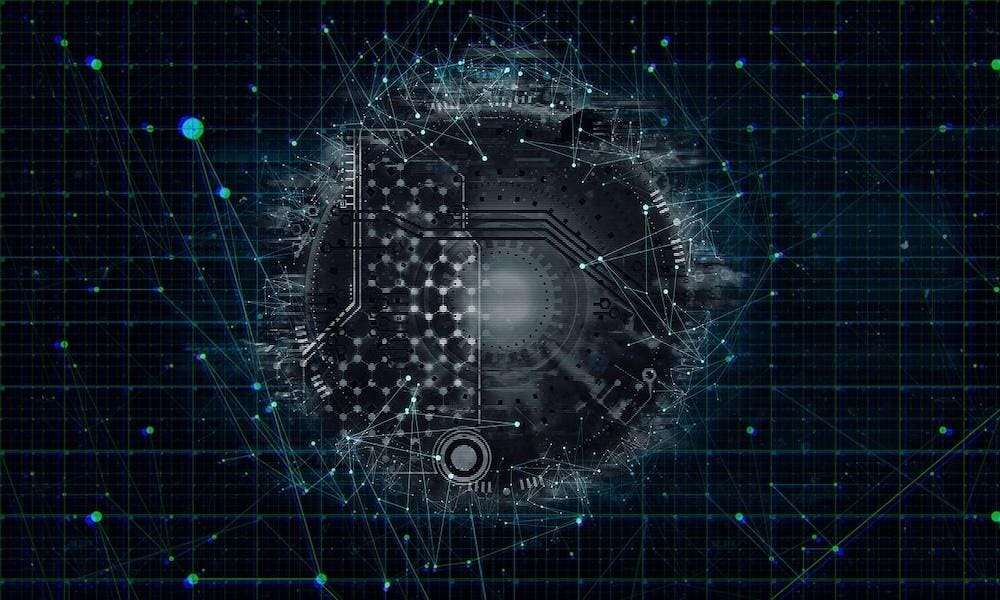
[ad_1]
Network
pixabay
With an emphasis on AI-first strategy and improving Google Cloud databases’ capability to support GenAI applications, Google announced developments in the integration of generative AI with databases.
The announcement highlights several key developments aimed at empowering developers and businesses to leverage the power of GenAI in conjunction with operational and analytical data systems.
Emphasis on AI-First Databases
Google’s vision for the future of databases includes a strong focus on AI-first capabilities and a commitment to deeply integrating technologies such as vector indexing and search. This approach is in response to the growing demand for databases that can seamlessly integrate with GenAI to enhance the development of AI-assisted user experiences. According to Google, 71% of organizations plan to use databases with integrated GenAI capabilities, highlighting the critical role of operational databases in the evolution of enterprise GenAI applications.
AlloyDB AI and Vector Search Capabilities
Google introduced enhancements to AlloyDB AI, making it generally available in both AlloyDB and AlloyDB Omni. AlloyDB is a fully managed PostgreSQL-compatible database optimized for GenAI workloads, offering superior performance for transactional, analytical, and vector workloads. The enhancements are designed to support enterprise-grade production workloads, enabling real-time and accurate responses for GenAI applications. AlloyDB Omni can run within the data centers and at the edge. AlloyDB AI is based on the pgvector extension for PostgreSQL. The integration of pgvector into AlloyDB allows users to work with vector embeddings, which are essential for building generative AI applications that leverage large language models. This integration facilitates the storage, indexing, and querying of vector embeddings directly within the AlloyDB environment.
Integration with LangChain and Vector Support
Google is also expanding its ecosystem support by open-sourcing LangChain integrations for all Google Cloud databases, including AlloyDB, Firestore, Bigtable, Memorystore for Redis, Spanner, and Cloud SQL for MySQL, PostgreSQL, and SQL Server. These integrations facilitate the development of context-aware GenAI applications by providing built-in Retrieval Augmented Generation (RAG) workflows.
Additionally, Google announced vector search capabilities across more of its databases, including Spanner, MySQL, and Redis, to aid developers in building GenAI apps with their preferred databases. With LangChain becoming the de facto orchestration framework for language models, developers can easily integrate Google Cloud’s managed databases into their workflow.
Vertex AI Integration
The announcement further highlights the integration of Spanner and AlloyDB with Vertex AI for model serving and inferencing using SQL, as well as the integration of Firestore and Bigtable with Vertex AI Vector Search. The goal of these integrations is to give GenAI apps semantic search capabilities. This shows how important operational data is for getting the most out of generative AI by giving users experiences that are real-time, accurate, and relevant to their situation across enterprise apps.
Google is not the only cloud provider to offer vector capabilities for its cloud-based managed databases. Its competitors took a similar approach to enable customers to generate and store embeddings in managed databases.
AWS offers a broad range of services for vector database requirements, including Amazon OpenSearch Service, Amazon Aurora PostgreSQL-Compatible Edition, Amazon RDS for PostgreSQL, Amazon Neptune ML, and Amazon MemoryDB for Redis. AWS emphasizes the operationalization of embedding models, making application development more productive through features like data management, fault tolerance, and critical security features. AWS’s strategy focuses on simplifying the scaling and operationalization of AI-powered applications, providing developers with the tools to innovate and create unique experiences powered by vector search.
Azure takes a similar approach by offering vector database extensions to existing databases. This strategy aims to avoid the extra cost and complexity of moving data to a separate database, keeping vector embeddings and original data together for better data consistency, scale, and performance. Azure Cosmos DB and Azure PostgreSQL Server are positioned as services that support these vector database extensions. Azure’s approach emphasizes the integration of vector search capabilities directly alongside other application data, providing a seamless experience for developers.
Google’s move towards native support for vector storage in existing databases simplifies building enterprise GenAI applications relying on data stored in the cloud. The integration with LangChain is a smart move, enabling developers to instantly take advantage of the new capabilities.
[ad_2]
Source link